The Sixth IASTED International Conference on
Computational Intelligence and Bioinformatics
CIB 2011
November 7 – 9, 2011
Pittsburgh, USA
KEYNOTE SPEAKER
Machine Learning Approaches for Systems Biology and Drug Discovery
Abstract
Recognition of cells, tissues and organs as complex systems with emergent properties has led to the creation of the field of systems biology, and this complexity has also been manifested in a number of prominent drug recalls due to unanticipated side effects. Cutting-edge machine-learning methods have an important role to play in understanding biological systems and aiding drug development. Cell imaging assays are widely used in drug development and systems biology, and improved methods to extract detailed information from imaging assays are needed. The CellOrganizer project provides tools for learning generative models of cell organization directly from images and for synthesizing cell images (or other representations) from one or more models. Model learning captures variation among cells and inputs can be two- or three-dimensional static images or movies. Current components of CellOrganizer can learn models of cell shape, nuclear shape, chromatin texture, vesicular organelle size, shape and position, and microtubule distribution. These models can be conditional upon each other: for example, for a given synthesized cell instance, organelle position is dependent upon the cell and nuclear shape of that instance. Major advantages of the generative model approach are that models learned from separate experiments can be combined into one synthetic cell instance, and that results from different microscope systems and different experimental conditions can be compared through the framework of the generative model parameters that describe them. This will be especially important for integrating results from diverse studies of the effects of drugs and other perturbagens. However, this leads to a second machine learning challenge. Since the number of proteins that can be affected is in the tens of thousands, and the number of potential therapeutics whose effects we would like to know is at least in the hundreds of thousands, exhaustive testing of all compounds on all proteins is not feasible. Active machine learning methods, combined with generative models, can provide a framework for exploring large perturbagen spaces to find potential therapeutics with high desired activity on a specific target while minimizing activity on other targets.Biography of the Keynote Speaker
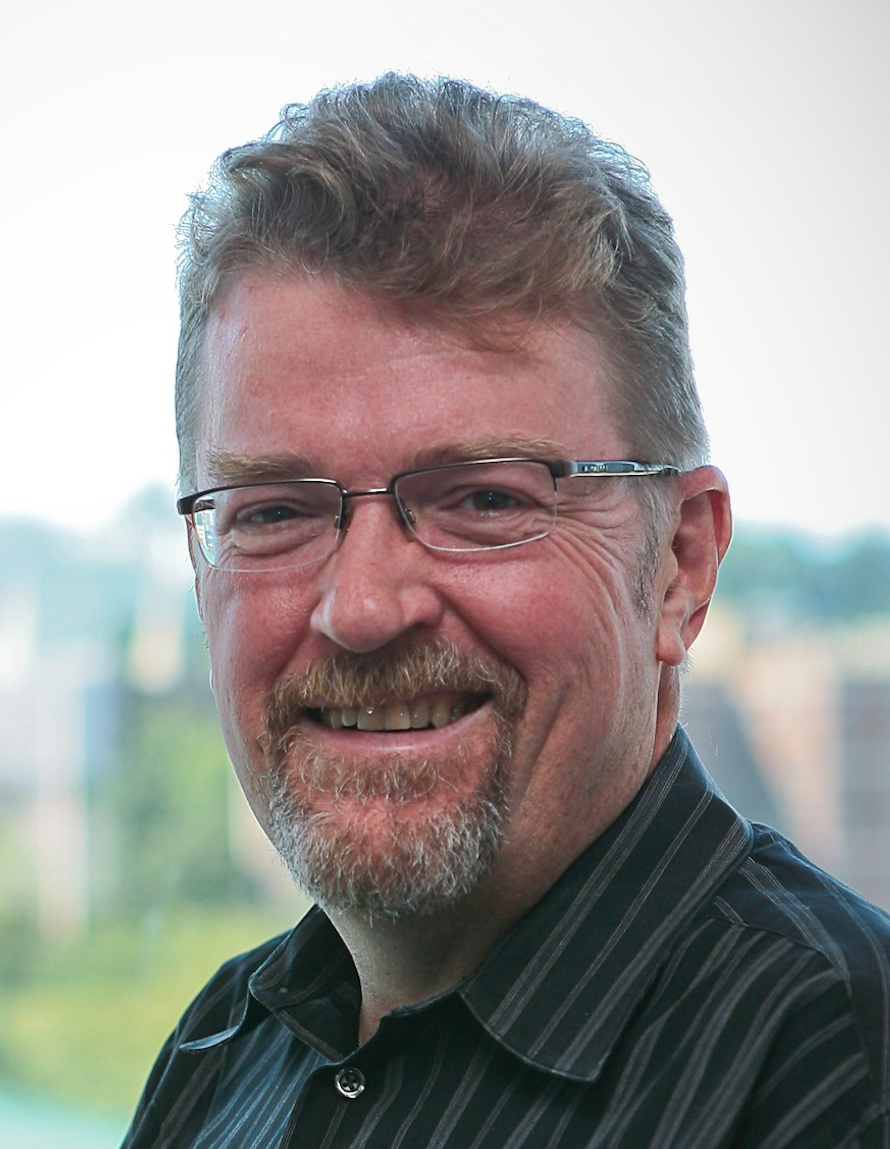
Dr. Murphy’s career has centered on combining fluorescence-based cell measurement methods with quantitative and computational methods. In the mid 1990’s, his group pioneered the application of machine learning methods to high-resolution fluorescence microscope images depicting subcellular location patterns. His current research interests include image-derived models of cell organization and active machine learning approaches to experimental biology.